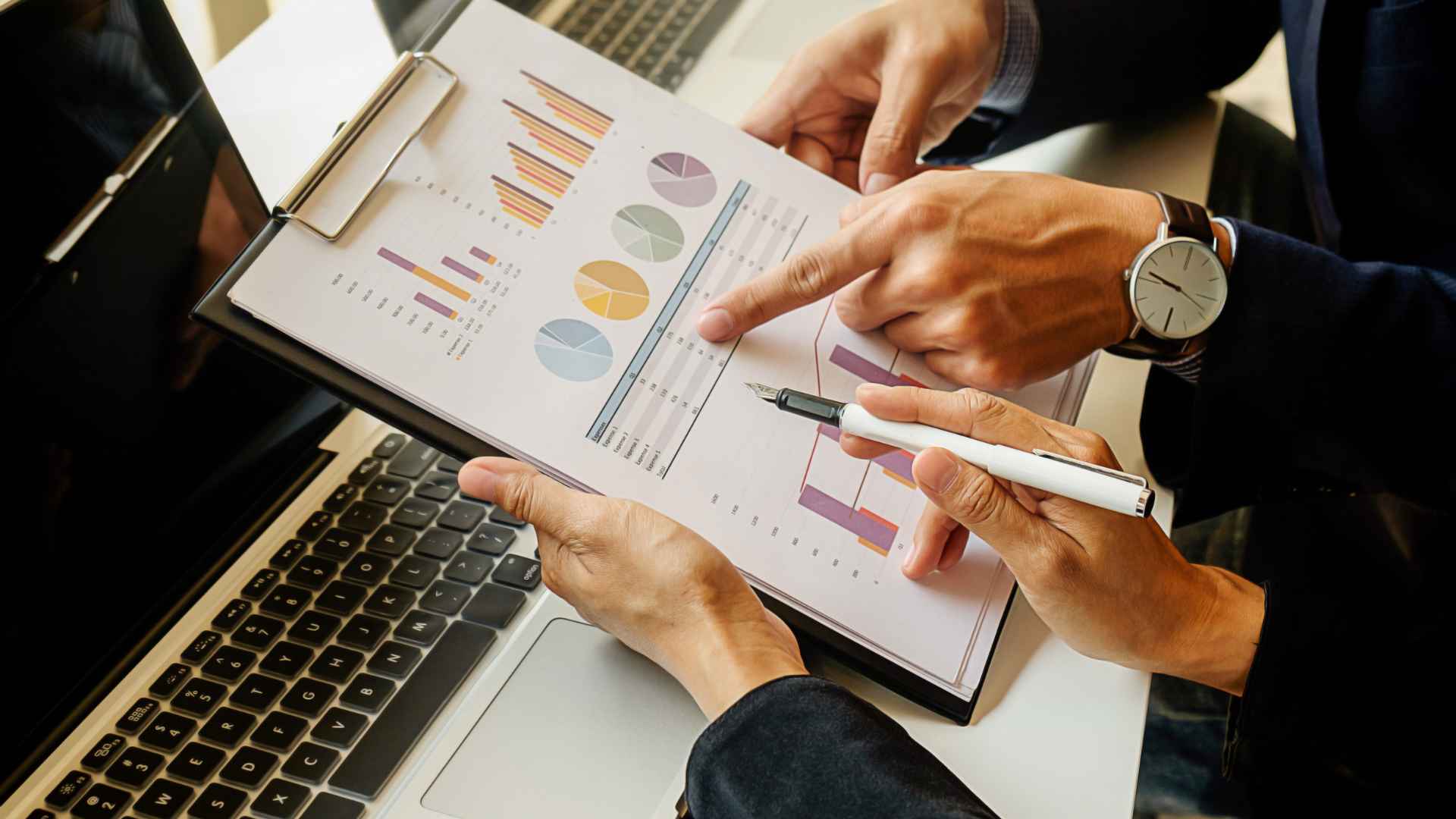
Imagine you are a financial analyst, and you need to create a financial model for a company or a project. You have to collect and analyse a lot of data, make assumptions and projections, and deal with uncertainty and risk. Financial modelling is a complex and time-consuming task, and you may not be confident about the accuracy and reliability of your results.
What if you could use the power of artificial intelligence to enhance your analysis and decision-making? In this blog, we will explore the integration of machine learning techniques into financial modelling and highlight real-world examples of how AI is improving forecasting and risk assessment. This way, you can navigate the intricate landscape of financial modelling with newfound confidence and precision.
The Marriage of Machine Learning and Finance
Machine learning (ML) is a subset of AI focused on enabling computers to learn from data and improve their performance over time. ML has been used in finance for decades, especially in areas such as algorithmic trading, credit scoring, and fraud detection. However, in recent years, ML has gained more popularity and attention in finance, thanks to the availability of large and diverse datasets, the advancement of computational power and algorithms, and the increasing demand for more accurate and efficient financial modelling.
Machine learning can offer many advantages for financial modelling, such as:
- Automating data processing and feature engineering: Machine learning can handle the complexity and volume of financial data and extract relevant and meaningful features from them.
- Enhancing predictive power and accuracy: ML can also assist in building more robust and flexible models that can capture the nonlinear and dynamic relationships between financial variables and adapt to changing market conditions and customer behaviors.
- Optimising financial decisions and strategies: Machine learning can optimise financial decisions and strategies, such as portfolio allocation, asset pricing, and trading.
Real-World Applications of AI in Financial Modelling
The use of AI in financial modelling is already transforming the field of finance. Here are some examples of how AI is applied in financial modelling in various domains and scenarios:
- Fraud detection: AI can help detect and prevent fraudulent transactions and activities by analysing large and complex datasets, identifying patterns and anomalies, and alerting the relevant parties. Financial technology company PayPal uses AI to monitor millions of transactions per day, and flag suspicious ones for further investigation.
- Algorithmic trading: By analysing large amounts of data, identifying market trends and opportunities, and optimising trading strategies and parameters, AI enables faster and more efficient trading than humans.
- Portfolio management: AI can help investors and wealth managers create and optimise portfolios, based on their goals, preferences, and risk profiles, by using recommender systems and generative AI. For example, Wealthfront is a robo-advisor that uses AI to manage investment portfolios for clients, by selecting the optimal asset allocation, rebalancing the portfolio, and minimising taxes.
Risk Assessment and AI
Risk assessment is the process of identifying, analysing, and evaluating the potential hazards and consequences of an activity, event, or system. It is a crucial component of risk management, which aims to reduce or mitigate the negative impacts of risk. Risk assessment can be applied in various domains, such as finance, health, security, and environment.
Improved Risk Assessment in AI
AI can revolutionise risk assessment in finance by using advanced techniques and tools to handle and analyse large and complex datasets, and to provide accurate and timely predictions and recommendations. It can also help financial professionals and organisations improve their risk assessment capabilities in areas such as:
- Credit risk: AI can help assess the creditworthiness of borrowers, especially those with limited or no credit history, by using alternative data sources, such as social media, mobile phone usage, or online behavior. AI can also monitor the repayment behavior and default risk of existing borrowers and provide early warning signals and intervention strategies.
- Market risk: AI can aid in measuring and managing the exposure and sensitivity of financial assets and portfolios to market movements, such as changes in prices, interest rates, or exchange rates. It can also help forecast market scenarios and stress tests and optimise hedging and trading strategies.
- Operational risk: AI plays a pivotal role in identifying and preventing fraudulent transactions and activities. By analysing extensive and intricate datasets, AI discerns patterns and anomalies, promptly alerting relevant parties to potential risks. Moreover, AI contributes to the automation and streamlining of processes and operations, mitigating human errors and enhancing overall efficiency.
The Role of Big Data in Financial Modelling
Big data refers to the large and diverse datasets that are generated and collected from various sources, such as financial statements, market data, customer data, social media, and sensors. Big data has a significant role in AI-driven financial modelling, as it provides the raw material for AI to learn from and make predictions.
The data acquisition and processing stages are essential steps for using big data in financial modelling. They involve the following tasks:
- Data collection: The initial step encompasses the collection and storage of data from diverse sources, ranging from databases and APIs to web scraping and cloud platforms. The gathered data may exhibit various structures, including numerical or categorical formats, as well as unstructured forms.
- Data Cleaning: Addressing the integrity of the dataset, this phase revolves around the removal or correction of incomplete, inaccurate, inconsistent, or irrelevant data. Techniques such as data imputation, outlier detection, or data normalisation are employed to enhance the dataset's quality and reliability.
- Data Transformation: This pivotal stage involves the conversion of data into a format conducive to analysis and modeling. Techniques like data aggregation, feature extraction, or feature engineering are applied, ensuring that the data is prepared appropriately for subsequent analytical processes.
- Data Exploration: Delving into the intricacies of the dataset, data exploration entails the analysis and visualisation of data to unveil their characteristics, distributions, and relationships. Employing methods such as descriptive statistics, correlation analysis, or clustering, this phase provides valuable insights to guide subsequent modeling and decision-making endeavors.
Challenges and Ethical Considerations
While AI in finance offers many benefits, such as improved accuracy, efficiency, and innovation, it also poses many challenges and ethical concerns, including:
- Data quality and availability: AI relies on high-quality and sufficient data to train and test the models. However, financial data can be noisy, incomplete, inconsistent, or outdated, which can affect the validity and reliability of the models. Moreover, financial data can be scarce, expensive, or inaccessible, especially for emerging markets, new products, or private companies.
- Model complexity and interpretability: AI can create complex and sophisticated models that can achieve high accuracy and performance, but at the cost of interpretability and transparency. Some AI models, such as deep neural networks, can be seen as black boxes that do not reveal how they make predictions or decisions, or what factors influence them. This can pose ethical, legal, and regulatory issues, especially in finance, where accountability is crucial.
- Model validation and evaluation: AI requires rigorous and robust methods to validate and evaluate the models and ensure their generalisability and stability. However, financial data can be non-stationary, heterogeneous, and dependent, which can violate the assumptions and requirements of some AI methods. Financial data can be subject to shocks, anomalies, and regime changes as well, which can affect the performance and validity of the models.
- Algorithmic bias and discrimination: AI can inherit or amplify the biases and prejudices that exist in the data, the algorithms, or the human decision-makers. This can lead to unfair and discriminatory outcomes for certain groups of individuals or entities, such as customers, investors, or borrowers. For example, AI can discriminate against people based on their race, gender, age, or income, when providing financial services, such as credit scoring, lending, or insurance.
The Future of AI in Financial Modelling
The future of AI and machine learning in financial modelling is bright and promising, as these technologies continue to evolve and innovate the field. Emerging trends include natural language processing, where AI processes and generates text, speech, or voice for applications like financial reporting and sentiment analysis. Additionally, computer vision and image recognition leverage AI to analyse and recognise images, aiding data visualisation and identity verification.
Finally, reinforcement learning and deep learning can enable the creation and training of intricate models capable of self-optimisation through learning from their actions and feedback. This narrative unveils a bright future where AI and machine learning reshape finance, fostering efficiency and innovative possibilities.
Become a Financial Modelling Expert with Nexacu
If you want to learn more about financial modelling, you can enrol in our Excel Specialist courses, particularly our Excel Financial Modelling Course for Beginners course. We will teach you how to build effective and robust financial models in Microsoft Excel. You will also learn the best practices and techniques for financial modelling, such as separating inputs, calculations, and outputs, avoiding hard coding, and using formulas and functions to perform calculations and analysis.
On top of that, you will also learn how to protect your models from undesired changes, and how to apply your skills to real business scenarios. We offer both remote and in-person classes, so you can choose the learning format that suits your schedule and preferences. Enrol today and transform your financial modelling capabilities with us at Nexacu.